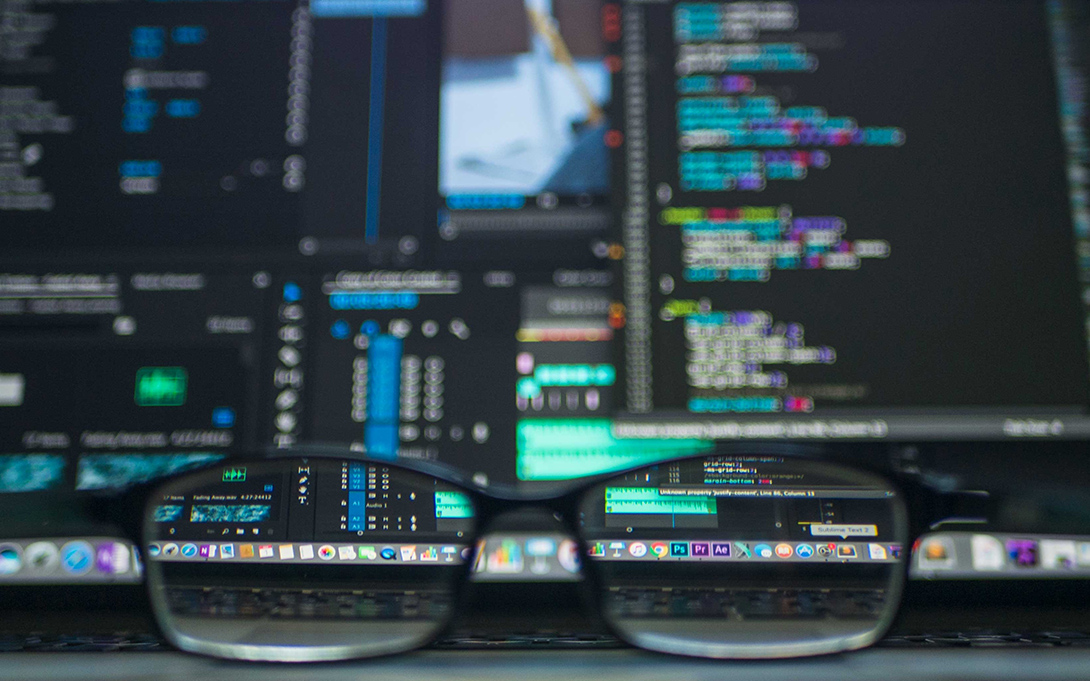
Large Language Models (LLM) — machine learning algorithms that can recognize, predict, and generate human languages on the basis of very large text-based data sets — have captured the imagination of scientists, entrepreneurs, and tech-watchers. While the technology can improve the effectiveness and efficiency of automated question answering, machine translation, and text summarization systems, even enabling super intelligent machines, some early studies have already suggested that the same shortcomings found in other types of artificial intelligence (AI)-based decision-making systems and digital technologies may also plague LLMs.
The Science, Technology, and Public Policy (STPP) program at the Ford School will explore the social, ethical, and equity dimensions of LLMs, thanks to a grant from the Alfred P. Sloan Foundation.
“We seek to develop a nuanced understanding of LLMs and their implications by using our novel analogical case study methodology. On this basis, we will produce actionable recommendations for LLM development, implementation, and governance. The key question is: How might we develop and regulate LLMs to maximize their societal benefits while minimizing their harm?” says STPP director Shobita Parthasarathy.
Parthasarathy has used the analogical case method as part of STPP’s Technology Assessment Project (TAP) since 2019. The method examines the social histories of previous technologies — particularly those that are similar in function or in their expected uses — and finds patterns in the development and implementation of emerging technologies to anticipate how they might transform the world. Developing such anticipatory insights is useful because it has the potential to inform technology design and regulation.
She, STPP Program Manager Molly Kleinman and a team of graduate student and postdoctoral researchers will systematically look at analogous technologies, including facial recognition, cyber currencies, and others, and also reach both far into the history of technology and across domains (e.g., to genomics and biotechnology) to identify social patterns that will likely guide LLMs and their impacts.
“What we have found in our previous work is that even the newest and most cutting edge innovations raise many of the same social and ethical questions as older generations of technology. Looking to those past cases can help us anticipate the impacts of technologies that people may say are too new to regulate,” says Kleinman.
On the basis of the analysis, they will provide recommendations not just for technologists but also for regulators, standards bodies, and publics, to help prepare for LLMs and their benefits while mitigating their harms.
The Sloan Foundation-supported study will produce a comprehensive, open-access report that anticipates the social, ethical, and equity impacts of LLMs based on analysis of the analogical cases; provide recommendations for LLM development, implementation, and governance; and produce a research paper about the study’s methodology.
“This case study analysis fits with the Sloan Foundation’s Trust in Algorithmic Knowledge program because it focuses on identifying the impacts of LLMs in a social context, with the ultimate goal of understanding how we should weigh the credibility and usefulness of knowledge produced by this innovative algorithmic technology. Its recommendations will provide concrete steps that scientists, engineers, companies, governments, and civil society advocates can take to manage the technology and its various applications, and will inform future areas of engagement by the Sloan Foundation and its grantees,” said Josh Greenberg, Sloan Foundation Program Director
The Alfred P. Sloan Foundation is a not-for-profit, mission-driven grantmaking institution dedicated to improving the welfare of all through the advancement of scientific knowledge. Established in 1934 by Alfred Pritchard Sloan Jr., then-President and Chief Executive Officer of the General Motors Corporation, the Foundation makes grants in four broad areas: direct support of research in science, technology, engineering, mathematics, and economics; initiatives to increase the quality and diversity of scientific institutions and the science workforce; projects to develop or leverage technology to empower research; and efforts to enhance and deepen public engagement with science and scientists.
More news from the Ford School