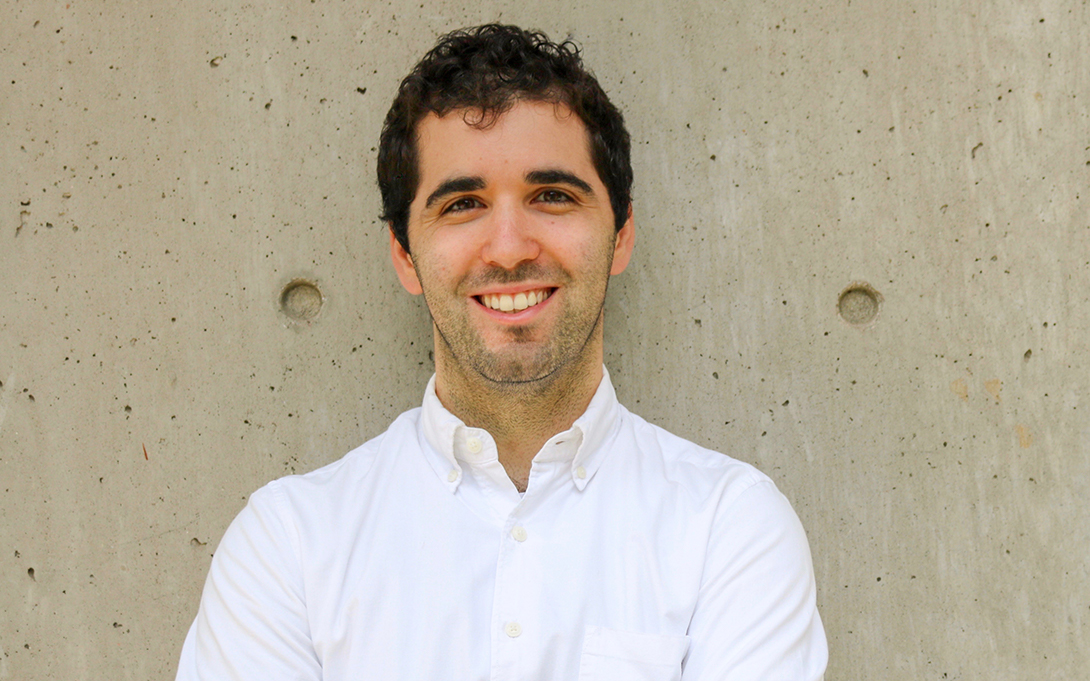
Machine learning is increasingly used for government decision-making to predict adverse outcomes. For instance, many cities and states in the U.S. have adopted pretrial risk assessments that inform decisions about whether to release criminal defendants before trial. A central goal of adopting algorithms is to improve decision-making by providing government staff such as judges with accurate risk predictions.
In a new study, however, University of Michigan Assistant Professor of Public Policy and Postdoctoral Scholar in the Society of Fellows Ben Green and computer scientist Yiling Chen (Harvard) provide the first direct evidence that algorithmic risk assessments can systematically alter how people factor in risk when making decisions and do not improve the quality of human decision-making. They demonstrate the unintended consequences of an effort aimed to improve public policy, but in fact creates more harm and exacerbates racial disparities.
With the goal to investigate how risk assessments influence decision-making processes, the study asked 2,140 U.S.-based participants to make decisions about either applicants for home improvement loans or felony defendants awaiting trial. Half of participants were shown the risk assessments, and the other half were not.
Green and Chen found that while risk assessments improved the accuracy of human predictions, they changed the way people factored risk in their decisions, which counteracted the benefits of enhanced predictions.
“In the pretrial setting, the risk assessment made participants more sensitive to increases in perceived risk, increasing racial disparity in pretrial detention by 1.9%,” they write. “In the loans setting, the risk assessment made participants more risk-averse at all levels of perceived risk, reducing government aid by 8.3%.”
The researchers warn that in practice, risk assessments can have unexpected and adverse consequences. They “generate unintended and unjust shifts in the application of public policy without being subject to democratic deliberation or oversight.”
The researchers conclude: “There is an urgent need to uncover potential issues in human-algorithm collaborations before algorithms shape life-changing decisions. Risk assessments are increasingly being integrated into high stakes decisions, yet consistently produce unexpected and unjust impacts in practice.”
The paper “Algorithmic Risk Assessments Can Alter Human Decision-Making Processes in High-Stakes Government Contexts” will be published in the journal Proceedings of the ACM on Human-Computer Interaction and will be presented at the 24th ACM Conference on Computer-Supported Cooperative Work and Social Computing in October 2021. Read it in its entirety here.
More news from the Ford School